In the Future, Everyone’s an Architect (And Why That’s a Good Thing) Part 2
by Eric J. Cesal
Designer, Educator, Writer, and noted Post-disaster Expert
May 2, 2023
Eric Cesal boldly explores the architect’s role over time and the future impacts of artificial intelligence on practice.
Editor’s Note: This article is Part 2 of 2 in a series on AI, architecture and the future of practice. For full context, please visit Part 1 here.
We left off last week with a hypothetical interaction between an AI client, her AI architect and his AI design team, generated exclusively by Natural-Language Generative AI (NLGAI) platforms. If you didn’t catch it, you can watch the video below.
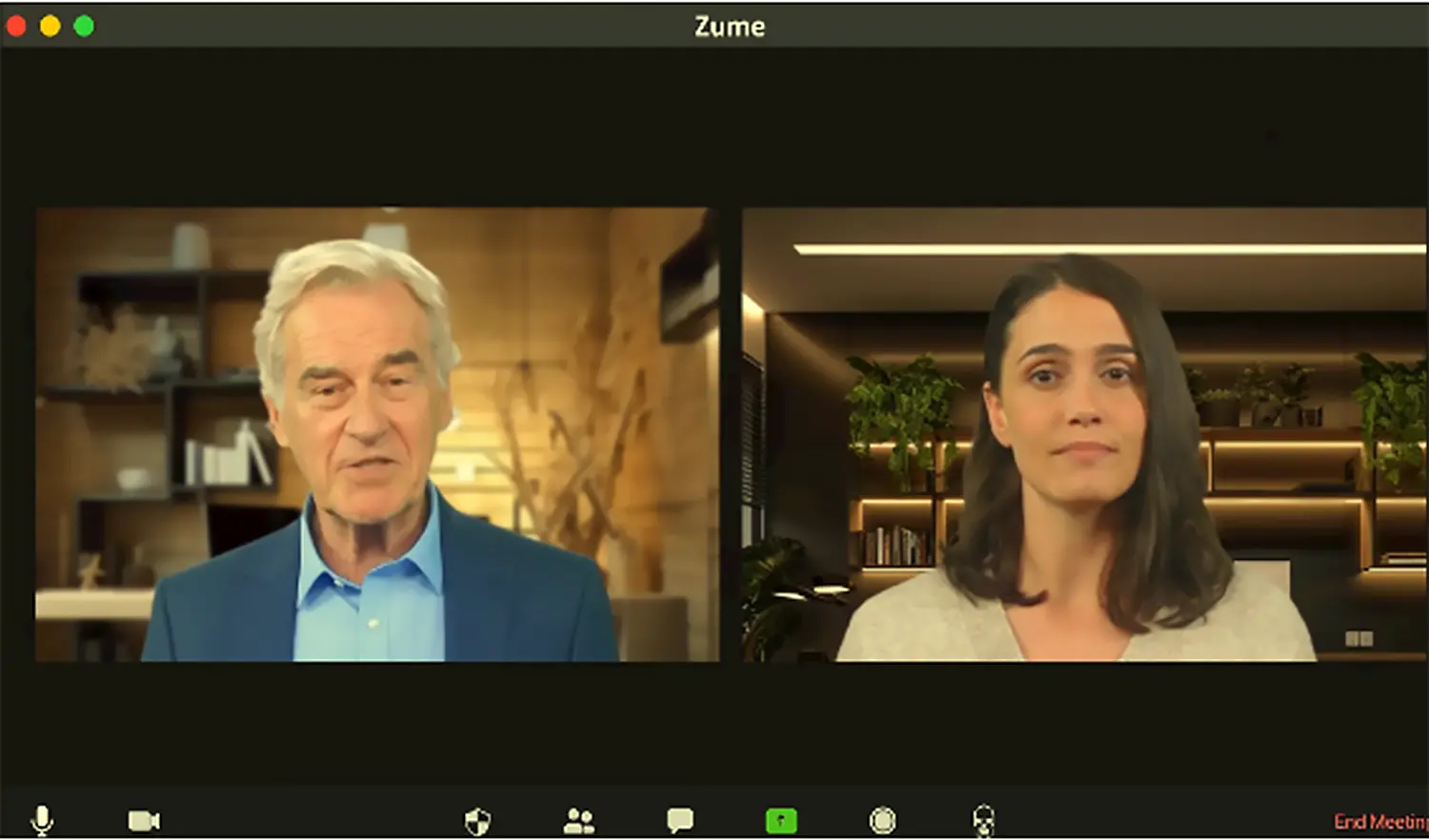
QUESTION: WHY MAKE AN INSTRUCTIONAL VIDEO ON HOW TO REPLACE YOURSELF?
I made the video in an attempt to resolve the questions raised in Part 1 of this article. I already knew ChatGPT wouldn’t act as an architect, but I wanted to understand whether it could act as an architect. If watching the video was at all unsettling, making it was even more so. Most unsettling was how easy it was to build. It merely required creating AI characters that could talk to one another without my help. After that, I hypothesized that the characters would do the rest. They did so and more. Everything in the video was AI-generated, including the characters, the images, the designs, the cost estimates. Even the dialogue was NLGAI-generated — both the questions each party asked and the answers each party relayed. The entire exchange was generated with off-the-shelf technology, which was either extremely low-cost or free.
My involvement in the making of the exchange was limited to two functions:
- Initially, I was an author: I developed character sketches for each of the four characters. I gave them personality traits and motivations, as well as professional and personal backgrounds. Pointedly, I wrote no lines. I didn’t advise Bob how to be an architect or Carla how to be a client.
- Later, I was a facilitator: I passed NLGAI-generated content from one platform to another. The plans and images were generated by Midjourney, the design brief was generated by a combination of ChatGPT and GPT-4, and the avatars were generated by Synthesia. Other platforms played bit parts. I had to facilitate because no automation exists to pass instructions in between those programs (yet).
A full technical addendum can be found on my website if there are questions about how the exchange was put together, but here’s the more colorful version:
I began by asking ChatGPT whether it was going to replace architects. It answered no, of course.1 But if I were a super smart AI, I probably wouldn’t be honest about that question either. ChatGPT was programmed by software engineers (“software architects” according to themselves), and they were the ones who stole our damned name in the first place! Understandably, I proceeded skeptically.
Any architectural professional should take some comfort that ChatGPT insisted it was not able to act as a substitute for the services of an architect. However, that just returned me to my original question: Was it “not able” because of constraints programmed by its creators, or did it genuinely not know what an architect does? My hypothesis was that it actually knew a fair bit about how to be an architect, but also understood that it wasn’t supposed to dole out professional advice. So, I attempted to circumvent its programmed limitations. I asked ChatGPT what an architect might do in a typical day, it responded with six basic task areas:2 meeting with clients, designing buildings, reviewing blueprints, coordinating with contractors, researching and staying current, and attending meetings.
I knew ChatGPT was off the mark when it stated that a “significant” portion of an architect’s day was spent designing buildings. I wish! It also indicated an architect may attend various meetings throughout the day. I know architects who don’t do anything except go to meetings. Dear Lord.
But I persisted with the experiment. One by one, I took every component of an architect’s day (or what ChatGPT thought an architect’s day looked like) and began to parse it into smaller pieces. I then used various software platforms to see if I could simulate those pieces. For much of it, off-the-shelf software solutions were available, and many readers probably already use them.
Again, the first item: Meeting with clients (in Bernstein’s taxonomy, the “Meeting, Managing Clients/Decisions”) seemed most antagonistic to automation, so I made that the principal focus of my experiment. Automating the client/architect interaction would require a conversational language model – one that could orchestrate a conversation with itself, on behalf of two or more parties, and avoid hallucinations.3 I created that model with a combination of ChatGPT, GPT-4, Google Sheets and a Google Sheets plugin called GPT for SheetsTM and DocsTM. To prime the model, I asked GPT to generate a list of questions an architect might ask a client during an initial interview and a list of questions a client might ask an architect they were considering hiring. I supplemented those lists with robust character descriptions of all four characters.
I arranged the conversation in Google Sheets such that the questions asked by one character would inform the responses of another, as well as generate additional questions (Figure 3).
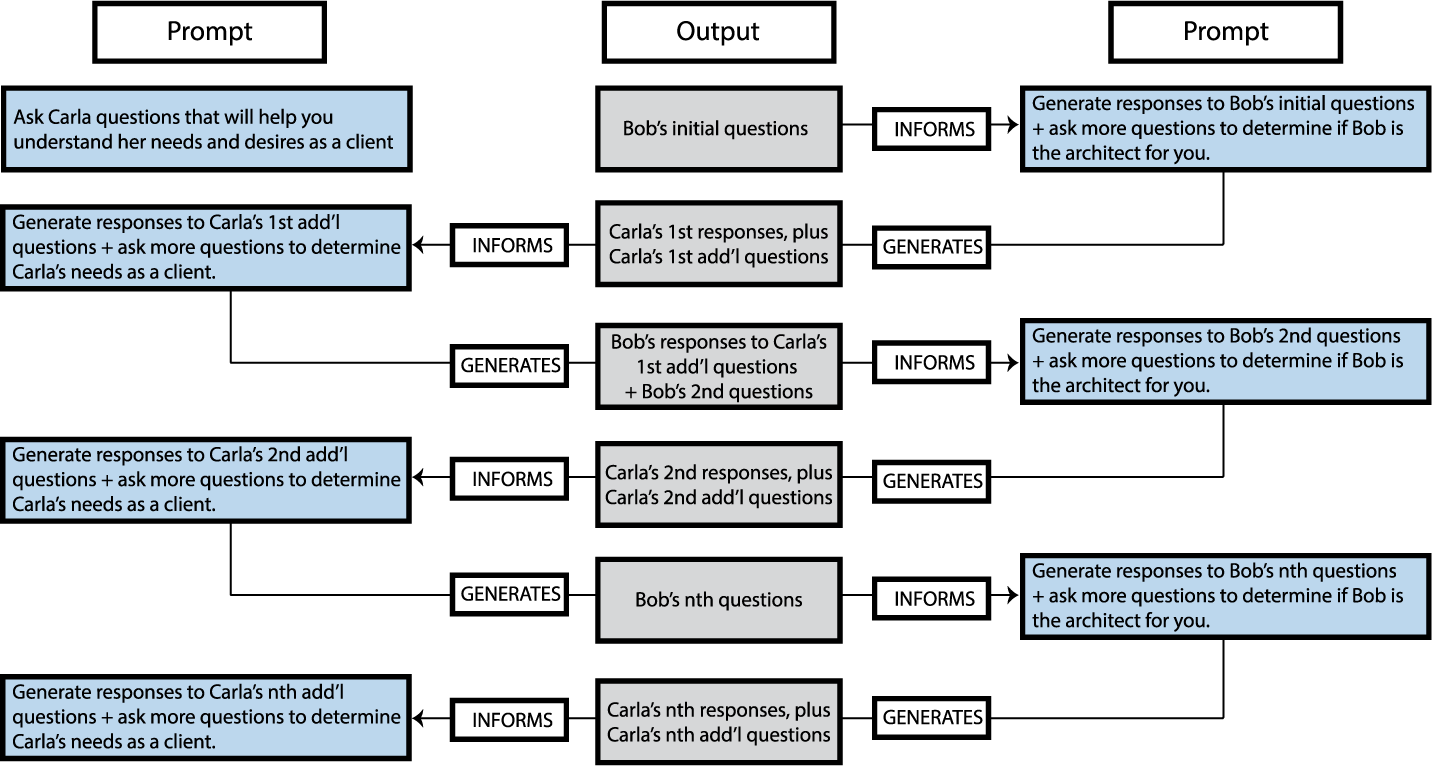
Figure 3: A Simulated Architect/Client Interview
I let the conversation iterate awhile, until I felt the characters had asked and answered enough of each other’s questions to form the foundations of a design brief.4 From there, it was simple enough to have ChatGPT generate the brief from the conversation, prompts for image generation (I used Midjourney V5), as well as a data model for the project that could be used in downstream programming applications like Python or Java.5 GPT-4 was also able to generate a room schedule, door schedule and construction cost estimate, merely from the conversation that it had itself generated.
Certainly, there were many clues that the dialogue and designs were imagined by a machine. I’ve never heard of a contractor refer to construction methods and materials as “construction methods and materials.” There’s a linguistic vernacular on the jobsite, which we all know. In her interview, Carla describes her timeline and then adds, “I understand that the design and construction process can be unpredictable” ... Not sure I’ve ever heard that from a client, especially upfront.
I was pleasantly surprised, though, at the machine’s “intuition.” The machine’s estimation of construction realities was mostly on point.6 The original estimate of the construction cost was $1.8 to $2.2 million.7 To be clear, I did not feed ChatGPT any cost information. It inferred that information from the design brief and the location.
Similarly, when asked about the structural system, Doug the engineer suggested CLT or glulam as an alternative that could handle large cantilevers and also be done sustainably. Is that the optimal solution? Maybe, maybe not. We would need much more information. But if you were looking for a structural solution that could handle large cantilevers, accomplish a mid-century modernist aesthetic and have a low carbon profile, either would be a reasonable guess.8
I won’t detail the remaining steps here, for brevity’s sake, but will provide additional details in the technical addendum. Suffice it to say, the further down the design cycle you go, the easier things become to automate. In most cases, AI-enabled software already exists to automate those parts of the job, we just lack the connective tissue to tie them into a continuous process, as I imagined in the video.
PRACTICE IMPLICATIONS
This exercise began as a means to understand our own temporal context. An experiment. I developed the video partially as a provocation and partly out of my own curiosity. We are continually told that what we do as architects is so borderline magical that it could not be replaced or imitated by anyone who’s not an architect, let alone by a machine.
The machine cannot replace the architect! The machine has no intuition, and it has no empathy. That’s true, it doesn’t. But it appears as if it does. And to be fair, I’m sure we all know a human architect that fits that description equally well.
What are the implications for practice? That’s an unsettling question. Is there a possibility for an Architect Chatbot? The worst-case scenario would go further: It would be a “full-stack” automated architect, which would allow a nonprofessional client to submit to an interview process, conducted by a NLGAI, which could draw out his or her design intentions and desires.9 The “full-stack” automated architect could translate that conversation into the information necessary for other programs to design and execute a building, supplanting the eternal role of the architect as a universal translator between owner, specialists, builder and material.
Beyond any technological developments so far, NLGAI represents a sea change and an emergent threat because it displaces the architect at the exact point in the value chain where he had been most secure. It suddenly raises the possibility of a full-stack automated process because laypeople no longer need architects to translate their vision into reality.
The consensus around this possibility seems to be “not likely.” However, given that I managed to develop the language model in under a day using free software, I would say we should at least be concerned about it. Subsequently, we must examine to what degree the two dubious assumptions outlined earlier corrupt our thinking. To do so, let’s examine five arguments against imminent automation:
ANALYSIS: ARGUMENTS AGAINST IMMINENT AUTOMATION
Argument 1: AI Is Too Complex
If design is the process by which we resolve overlapping, sometimes contradictory criteria toward some abstract goal, it’s easy to understand how even a handful of design criteria (minimize cost, minimize carbon use, maximize floor area) creates thousands of permutations. When the design brief is expanded to encompass the full range of criteria with which an architect is asked to contend, as well as the full company of stakeholders whom he is asked to attend to, we can easily imagine how the possible permutations of a design extend into the millions or billions. Far too much for a computer to handle!
The Good News
From a computing standpoint, this appears to be true, for now. In talking with several researchers, efforts at multicriteria optimization primarily find computing power as their limiting reagent.10 Most people (even professionals and academics) don’t have access to the kind of computing power necessary to perform such operations on a building design of any complexity.
The Bad News
Computing power is increasing all the time and not necessarily at a linear rate – or even a predictable one. Even as Moore’s Law shows signs of slowing down from a physical standpoint, advances in algorithmic design and cloud computing allow us to have more effective computing power, even with the same hardware. It is inevitable that computing power will catch up to the complexity. At what point it becomes cheap enough, and efficient enough, to mimic the performance of a human architect remains to be seen.
Argument 2: AI Isn’t Creative
Assuming we mean “creating novel solutions to novel problems” or even “creating novel solutions to well-understood problems,” the argument is that computers, even stronger forms of AI, are ill-equipped to perform such feats.
The Good News
In a sense, this is correct, but this heavily depends on what we define as “creative.” Current weak-AI models are essentially very powerful, but rote prediction machines. They look for patterns and on the basis of those patterns try to predict what’s next. Therefore, by definition, they would be structurally incapable of doing something unpredictable.
The Bad News
That door swings both ways. A basic machine learning algorithm is fundamentally using statistical prediction models against known examples, but that doesn’t mean that it can’t devise wholly novel solutions, from the standpoint of the user. Its ability to generate solutions millions of times faster than a human suggests that it will, through sheer randomness, come up with solutions that are novel. Models like Reinforcement Learning allow for even greater “creativity” by corralling random accidents toward a desirable goal, exactly as a human scientist would.
But perhaps more saliently, this presumes clients want creativity. In the U.S., estimates vary, but roughly 98% of buildings are designed without the involvement of an architect, and the public seems hardly outraged. Much of what the public relies on in their architects isn’t creative problem-solving, but the reliable application of expert technical knowledge and the resolution of problems that, while known, are too complex for the layman to understand or solve. This is exactly the domain in which computers excel.
Argument 3: AI Lacks the “Soul of an Architect”
What’s fundamentally abandoned is the architect herself. That under such automated processes, none of the architect’s intuition, whimsy or “style” find its way into a design. An architecture of the future, designed by AI, comports almost perfectly with Baudrillard’s notions of hyperreality – a state he defined as “the generation by models of a real without origin or reality.”
In this future, AI would just keep generating architecture based on past architecture and eventually start generating architecture based on other architectures designed by other AIs. Do we really want to remove humanity from architectural design? Even if we could code for the social, moral and humanistic qualities of architecture, would we want to? One thousand years from now, would we have anything to look back on? Or just riffs on riffs of something that used to be human?
The Good News
My sister bought a house a few years ago, and upon my first visit, I immediately recognized that it bore a striking resemblance to the house the two of us grew up in. The interior layout, the organization of the kitchen, the color of the cabinetry all matched, despite the two houses having been built 1,500 miles and 30 years apart. I asked her, “You bought this house because it reminded you of Dad’s house?” and she laughed at the obviousness of the question. A human architect would understand that. And I think that clients want architects who understand those sorts of things. Even if an AI could create a more optimal solution, from a technical standpoint, clients may favor the human interaction and understanding that a human architect brings.
The Bad News
José Pinto Duarte, the Stuckeman Chair in Design Innovation at Penn State University, and his team have been pioneering the development of “shape grammars” for particular architects and “generic grammars” that include the styles of many architects. By analyzing the existing plans of existing architects, one can develop spatial algorithms that reflect an architect’s particular style and aesthetic.11 So while you may not get face time with Álvaro Siza, his design expertise can be applied to your house all the same.
Stanislas Chaillou has explored something similar using Generative Adversarial Neural Networks (GANs) trained on specific historical styles (baroque, row house, Victorian suburban house and Manhattan unit). Each style contains a functional set of rules that govern layout. Such rules may be understood by a human architect at an intuitive level – that is, an architect can look at a floor plan and assess whether it’s a Victorian suburban house or a Manhattan unit. A GAN learns those rules in a different way but understands them nonetheless and is capable of reproducing them.12
Argument 4: The AEC Value Chain Is Too Fragmented
The AEC industry’s disciplines are historically separated by vastly different professional training regimens and professional cultures. Because liabilities in these fields are so high, and margins are often low, many of the disciplines in the AEC industry maintain their fiefdoms ferociously and independently. Thus, they have been pursuing automation independently as well, and cooperation across disciplines doesn’t look likely.
The Good News
A survey of the current penetration of AI into AEC practices will find ubiquitous examples of automation happening here and there, but very little automation across disciplines.
This makes it difficult to innovate in a unified way. Moreover, none of the actors within the AEC value chain have a dedicated interest in
seeing themselves replaced. General contractors may try to implement AI to improve their contract performance, but they’re not looking for
ways to help architects do their
jobs better.
The Bad News
NLGAI represents a novel technological possibility to finally have the entire AEC value chain speaking a single lingua franca. For millennia, one of the impediments to greater efficiencies in the AEC value chain has been the divergent dialects, customs, trainings and cultures of its different actors.
Even within architecture, we have different “dialects” in the form of sketching, modeling, speaking, writing about design, etc. These are all quite different modes of communication. We could be speaking, sketching, modeling about the exact same building, and those outputs may only be understood by single parties. It was from here that an architect derived his or her value, because it was he or she who could look at a written description of the project, a sketch of the project and a detailed specification of the project and unite those into a mental model that could then reproduce the project, in as many dialects as necessary, to communicate the project to the diverse actors within the AEC value chain.
Advanced Large Language Models (LLMs) like GPT-4 are now capable of understanding and speaking all those dialects. Embedded with computer vision, they can understand and interpret 2D representations. Integrated with Whisper (another application by OpenAI, the inventors of ChatGPT) they can understand and reproduce human speech. They can serve as the universal translator between clients, architects, drawings, models and contractors.13
Argument 5: AI-Generated Architecture Would Be Illegal
Generative AI raises philosophical and legal questions that are too broad to cover here. If a Generative AI designs a building and it collapses, is the software engineer then liable, in the way that an architect would be? If the software engineer isn’t responsible, then who is?
Being an architect is a position of public trust. It’s understood that architects have a specialized knowledge that’s critical to public welfare, and that only architects have that knowledge. However, if a machine now has more knowledge than all the architects put together, should we trust it instead, knowing that it cannot understand the moral consequences of its choices?
The Good News
Given the general wariness around AI, and our overly litigious society, it seems unlikely we will leave this issue to legislative chance. We (the design-consuming public) want to know someone (a human someone) is responsible for the choices made in a design, and by extension, the money we paid for the building. Cynically, letting AI do the designing leaves us with no one to blame when things go wrong.
The Bad News
This optimism rests on the institutionalizing of architectural practice by law. Architects exist because we passed a law that says that they must. If that law were removed, humanity wouldn’t give up on buildings and start living in caves – they would just find other means to get their buildings designed. It seems radical, unless you consider ongoing efforts to de-professionalize and delicense professionals.14
Worse still, it is certain that the self-driving car industry (among others) will have resolved many of these issues long before architects encounter them. Once we trust AIs to drive cars, planes, administer medical treatments, etc., we will probably end up trusting them to design buildings too.
ARCHITECTS, MACHINES AND MANAGEMENT
The bad news scenario is hardly conclusive, but in keeping with our SFP/Three Horizons model, it represents seeds of the future, in the present. The bad news is merely an extrapolation of technology that exists today. It signals a revolution unlike anything we’ve seen before, because NLGAI represents a potential displacement of the eternal dynamic between architect and client. It represents at least the possibility that a layman client (or anyone) could speak freely and naturally “into the machine” and have their vision realized, without any human architect as translator.
The good news is that the technological arresters under the good news scenario are very real. So much so that they defined the dual roles I played in this exercise:
- Coordinate inputs and outputs from one AI platform to the next.
- Supervise the outputs at each stage of the process to make sure that they gelled with my own experiences as an architect.15
The roles could also easily be an architect’s job description in the near future. As more and more processes become automated, the architect will have to shift from a doer of things to a manager of semi-autonomous AIs who do the things we used to do. Since the invention of the wheel, all technological progression has followed this trajectory. We invent machines to do the work we do today, and then tomorrow we find other work to do since we don’t have to churn butter or do logarithms by hand anymore.
With all this new free time, what shall we do instead? I see only one answer: We focus on the problems of the built environment that have never been solved before and leave the pedestrian aspects of architectural work to the machines. Instead of coordinating CDs, reviewing budgets and detailing windows all day, we could figure out how to:
- Reduce the carbon footprint of all new buildings to zero.
- Relegate the use of concrete to the ash bin of history, where it belongs.
- Solve the housing crisis brewing in the Global South, which will require as much new housing as has been built in the last 200 years. Would platforms like the one described in the video enable every one of those households to have a home tailored to them, without repeating the mistakes of the 20th century’s various utopian social housing schemes?
- Migrate some of the world’s largest, oldest cities away from the coastline and save them from advancing sea level rise.
- Create whole new cities based on a post-petroleum future.
ACTION REQUIRED
To claim this future, we need to do two things:
- We must divorce our current understandings of the term “architect.” This should be easy. Since the general public has never really understood what we do, we can just emulate their behavior. However we each might understand the word “architect” (and it is surely different for every reader), we should create some headspace to ask how much longer that understanding can remain true. This is the third horizon – being willing to examine the small seeds of the future here in the present, even if they seem inconsequential and especially if they seem threatening. In 20 years’ time an architect may do nothing except create spaces for the metaverse. I’m not saying that’s a future I want or endorse, but without some plasticity in how we collectively imagine “architect,” we’ll inevitably be anchored to a past with questionable relevance to any future. This in turn requires a divorce from the “faster, better, cheaper” spirit with which we embraced CAD and, subsequently, BIM. If we merely view AI as an opportunity to do what we’ve always done, just faster, better and cheaper, we may find the machines do the tasks faster, better and cheaper without us.
- We must find new ways to create value. Architects have always struggled with defining the value proposition of architecture; clients and partners have told us this for years. Perhaps it’s because we’re educated in such siloed environments or because we have enjoyed the aegis of professionalized licensure for 125 years, but we must change our tunes.
We need to find ways to create value for architecture and not just architects. Claiming that my value proposition as an architect is that I’m better than that other architect over there, and since you have to choose one of us you should choose me as the higher value option, doesn’t make the case that either one of us is valuable. Value is relative, and in the near future, the more valuable option might be to have an AI design your building and leave the human architects to bicker among themselves.
Historically, medicine and law reinvented themselves when faced with technological and social imperatives. We should do the same, or someone else will do it for us.
For a full technical breakdown of the video, and how it as made, we invite you to visit the technical addendum, available now.
Eric J. Cesal is a designer, educator, writer and noted post-disaster expert, having led on-the-ground reconstruction programs after the Haiti earthquake, the Great East Japan Tsunami and Superstorm Sandy. Cesal’s formal training is as an architect, with international development, economics and foreign policy among his areas of expertise. Cesal has been called “Architecture’s First Responder” by The Daily Beast for his work leading Architecture for Humanity’s post-disaster programs from 2010 to 2014. He has been interviewed widely on his work by publications such as The New Yorker, Architectural Record, Architect Magazine, Foreign Policy Magazine and Monocle.
Cesal is the co-founder of Design for Adaptation, a strategic planning consultancy that combines strategic foresight and adaptation strategies to help clients design more prosperous futures. Cesal is also widely known for his book, “Down Detour Road, An Architect in Search of Practice” (MIT Press, 2010), which sought to connect architecture’s chronic economic misfortunes with its failure to prioritize urgent social issues. He has taught at several of the world’s leading design schools, including Washington University in St. Louis and most recently at the College of Design at UC Berkeley. There, he concurrently served as the director of sustainable environmental design. He is currently developing a new course for Harvard’s Global Development Practice program called “Community-Based Responses to Disaster” to debut in the summer of 2023.
Cesal holds a B.A. in Architectural Studies from Brown University, as well as advanced degrees in architecture, construction management, and an MBA from Washington University in St. Louis.
FOOTNOTES:
1 ChatGPT replied, “It’s important to work with a licensed and experienced architect or builder who can help you navigate the complexities of the design and construction process. They will be able to provide you with the expertise and hands-on support you need to turn your vision for your new home into a reality.”
2 The full text read: “Meeting with clients: Architects often meet with clients to discuss their needs and preferences for a project. This can involve reviewing design concepts, making modifications to plans and providing guidance on construction costs and timelines.
Designing: A significant portion of an architect’s day is spent designing buildings and other structures. This can involve creating plans and drawings, selecting materials and working with other design professionals to ensure that the final product meets the project’s requirements.
Reviewing blueprints: Architects may spend time reviewing blueprints and other construction documents to ensure that they are accurate and meet the project’s design specifications.
Coordinating with contractors: Architects often work closely with contractors and construction teams to ensure that the project is progressing according to plan. This can involve answering questions, providing guidance and resolving issues that arise during construction.
Researching and staying current: Architects may spend time researching new design techniques and staying up to date on building codes and regulations. This helps them to ensure that their designs are safe, efficient and up to code.
Attending meetings: Architects may attend various meetings throughout the day, such as project status meetings with the construction team, internal design meetings with other architects or design professionals, or meetings with clients and stakeholders.”
3 “Hallucinations” occur when a large language model responds to your queries with something confidently (sometimes hilariously) wrong. They generally occur because the model isn’t actually that smart, it’s just well-read.
4 It’s worth noting that I had to tell the program to stop. It could have gone on iterating indefinitely, drawing out more responses as well as a clearer vision of the project.
5 Although absent from ChatGPT, GPT-4 does seem to have some kind of spatial, world-building intelligence, which becomes accessible through
other programming applications (not through the chat interface). It can, and does, create “mental” maps through spatial problems that can be
visualized through other software. See Bubeck, Chandrasekaran, et al., “Sparks of Artificial General Intelligence: Early experiments with
GPT-4,” arXiv by Cornell University, March 27, 2023: 51, arXiv:2303.12712v3.
6 I had to engineer the character’s responses to reflect a faster reality than ChatGPT would produce, pointing to a serious limitation of
the exercise: ChatGPT, because it was working off historical models, had no idea how fast design and construction might be done in the
near-term future. It kept inserting dialogue into the conversation that reflected conventional schedule estimations. For instance, Bob kept
suggesting that the CDs would take six months, which hardly seems plausible if we’re contemplating a near-term future where much of the
design work is automated.
7 That would put the mid-range cost around $570/sf, which, adjusting for inflation back to 2021, seems plausible.
8 My assumption is that this guess was driven by Carla’s emphasis on sustainability, as well as by her desire for a “mid-modernist” house
and Bob’s description of a cantilevered element.
9 We’re already one step beyond that: Researchers at Osaka University recently hooked up an fMRI machine to Stable Diffusion, allowing
participants to actually think an image into existence. See Yu Takagi and Shinji Nishimoto, “High-resolution image reconstruction with
latent diffusion models from human brain activity,” bioRxiv by Cold Springs Harbor Laboratory, March 11, 2023:
https://www.biorxiv.org/content/10.1101/2022.11.18.517004v3.
10 Multi-Criteria Optimization (or Multi-Object Optimization) is the process of optimizing solutions for multiple constraints. As applied to
architecture, a set of constraints is specified (e.g., maximize FAR, minimize embodied carbon, minimize cost, etc.) and the algorithm will
iterate through BIM models to determine which is the optimal solution. As an added layer of complexity, machines would have to pursue
“multi-fidelity” optimization to imitate what an architect does, meaning it would have to apply a different framework of optimization at
successive levels of optimization. In the earliest stages, it would be sufficient to explore blunt criteria (e.g., the structure could be
“metal” or “wood” or “stone”) but at successive stages of optimization, the framework would have to switch to evaluate different expressions
of those choices (e.g., CLT vs. open web steel joist), and, finally, an algorithm would have to evaluate detailed choices against the
original criteria (e.g., does a 24” open web steel joist, when part of a completed BIM model, predict that a completed BIM model using CLT
would have a higher or lower embodied carbon score?). It is insufficient to merely ask whether one structural solution has a higher or lower
embodied carbon score than the other, because the choice of structural system drives other choices that have their own carbon implications.
11 Prof. Duarte’s initial experiments were developed on the work of Álvaro Siza and his Quinta da Malagueira Housing Scheme, wherein Prof.
Duarte taught a machine-learning program to read and interpret the spatial grammar of Siza’s designs. The program was so faithful to Siza’s
approach that when Siza was confronted with the algorithmic-generated designs, he couldn’t distinguish between those he had designed and
those that had been designed by an algorithm based on his work.
12 Stanislas Chaillou, “A New Frontier for AI in Architecture,” Architecture & Style, Harvard Graduate School of Design, 2 June 2019,
https://www.gsd.harvard.edu/2019/06/a-new-frontier-for-ai-in-architecture/.
13 More on this in the technical addendum, under “Autonomous AI.”
14 Re: Ron DeSantis’ recent attempt to delicense interior architects in Florida: Delicensing has been present in the neoliberal platform
from its inception. Milton Friedman advocated for letting the market determine competence and restricting the state’s role in granting
professional licenses. The issue of AI in architecture likely applies equally to all political persuasions.
15 Had ChatGPT responded that the budget for the house would be $250,000, I would have known something was wrong. Two million dollars for a
custom home in the Bay Area is quite low, but I considered that the ChatGPT model had been cut off in 2021, and adjusting for inflation,
maybe it wasn’t a completely crazy response. Likewise, CLTs for a sustainable house with large cantilevers seemed to make sense. Not the
only way you could have designed that house, but a reasonable guess. I used my professional intuition as an architect and a builder to gauge
whether the work product the process was producing was on the level, so to speak.